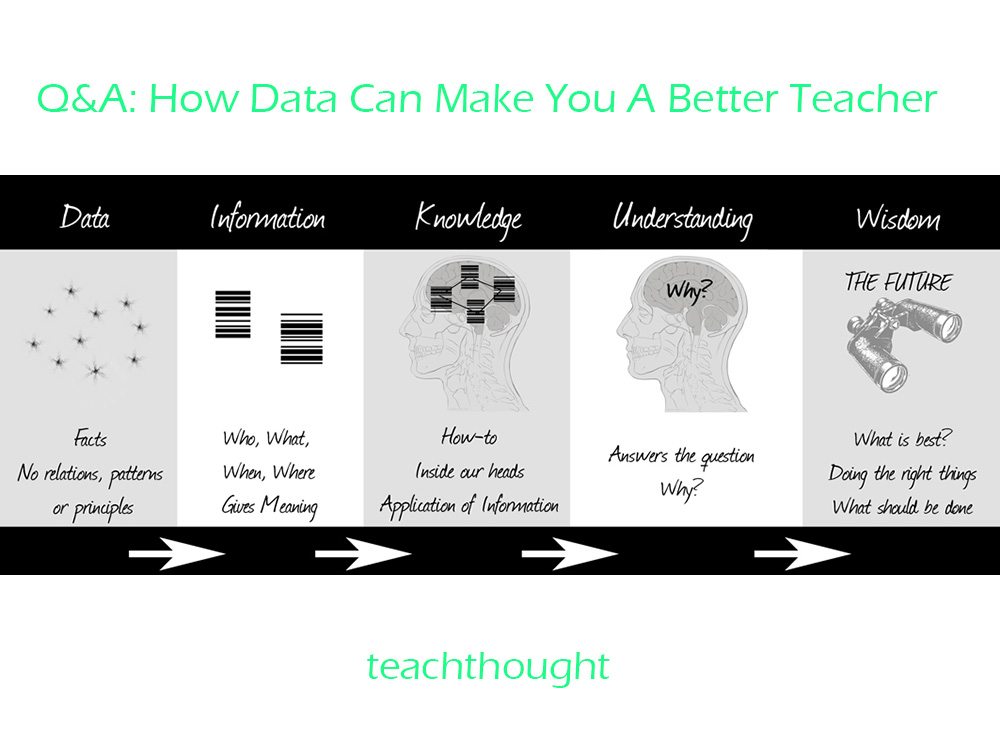
Q&A: How Data Can Make You A Better Teacher
by TeachThought Staff
Students today are more industrious and connected than ever.
They engage other students and professors in online forums; they autonomously seek and use personalized learning applications; and they leverage textbook supplements all in service of getting an edge, and trying to make the grade.
This Q&A seeks to answer what visibility instructors have into student engagement, and explore how they’re actively leveraging student data to become more effective teachers.
Andrew Smith Lewis is the Co-founder and Executive Chairman at Cerego. Barbara Illowsky is a Professor of Mathematics & Statistics at De Anza College. They are collaborating on a grant from the Bill and Melinda Gates Foundation to transform education for at-risk and underserved students. Below is a transcript of an interview between Andrew and Barbara.
Andrew Smith Lewis: What types of learning applications, tools, and services do students use these days and how do these tools impact student performance? What types of services and tools would you like to proliferate?
Barbara Illowsky: Students, especially lower-division undergraduate students, want fast, easy and self-directed learning. They expect immediate feedback, whether it is during a face-to-face (f2f) class or 3 o’clock in the morning. Gone are the days when a student learns three weeks after a due date or exam how well s/he performed. Gone are the days when a student waits for the next class or an office hour or tutoring center opening to get assistance or confirmation. Gone are the days when students sit for hours pondering the same content over and over again until they are convinced they have mastered it. And, in my opinion, good riddance to those former ways of teaching and learning!
Most importantly, students want many forms of online supplements available to them so that they can choose what fits their needs. Students use tools that they can access immediately. Most students carry a cell phone and/or tablet. Their devices are often the first place they access to find information such as the text, simulations, homework systems, and even counseling appointments. They want their instruction in small chunks with interactive formative assessment build in.
As soon as students are “stuck” on a problem, for example, they want to find a very similar example to review so that they can go back to the original problem. If they forget the steps they need they want to find that information on their devices. They use tools that verify their knowledge and fill in the gaps, when necessary. I give students the option of doing their homework online or with paper and pencil. Almost every student wants the online version so that they can get immediate feedback and similar problems to review.
ASL: How do you define and evaluate student success, “engagement,” and overall course efficacy? What data points or signals do you consider? What has proven most valuable?
BI: The measurable student success is the student earning the grade that s/he has strived for via demonstrating to me sufficient knowledge of the content. From my perspective, success can be displayed via alternate ways. Statistics and mathematics are often challenging courses for students. When a student finally succeeds in a small task, I see student success, even if that student does not complete the course. When a student realizes that s/he needs assistance and accesses optional learning materials, that is a success, too.
I teach both online and f2f classes. Traditionally, faculty have measured or recorded engagement as mostly in-class participation, participation in online discussion threads, and completion of assignments. Yet, engagement, even in f2f classes has dramatically changed with the advancement of supplemental learning apps and devices. Students may be shy in class, but leaders online. Students might not verbally ask questions yet search for answers and participate in online activities, both learning and assessing, solo and with others. Savvy students are finding ways to direct their own learning and not rely solely on the instructor and traditional class. This process is even more widespread in online classes.
One of the ways I measure engagement is via analytics of the online student support built into my courses. However, I know that I am not truly getting the full picture of how my students learn. I need personalized data informing whether students have accessed the support that they need, not what I necessarily provide. I need to know what learning apps would help them and be able to provide that support, too.
What has proven most valuable for assisting me in increasing student learning has been the addition of the WebAssign learning system into my courses.
ASL: Knowing what you use, what types of data would you like to have? In other words, what untapped student data, or application “exhaust” data, would you find most valuable, and why?
BI: I would love to know how long a student actually spends with a learning application and/or working on problems and what they are doing during that time, such as switching between support sites, working on a calculator, reviewing the text. These data are different than how long the student was logged onto the site or how long a video was playing. I would like the dedicated time. I would find it useful to know what about certain concepts make them difficult to learn. Is it the concept or the foundational background needed that is the challenge?
When attempting assessment, whether graded or not, I would like to know whether they needed practice problems and supplemental resources, how useful those additional resources are and why, and what else they needed.
ASL: How, if at all, do you generate student feedback loops around new applications, tools, or services? How do we know it’s working? How much does student feedback weigh into your decision to continue (or cease) using these applications, tools, or services?
BI: One of the challenges that faculty and academic researchers have when studying “interventions” is in getting control versus experimental groups with limited outside variable interference. As a faculty member, if I am teaching two sections of the same course the same term, even with an intervention in one section but not the other, it is still difficult to make statistically accurate conclusions on the effectiveness of the intervention.
I survey my students and do short assessments about all applications. I also ask open-ended questions allowing students to provide feedback. For example, about a third and then two-thirds of the way through the term, I give students the following four questions, due a week later and worth points.
- What is working well for you in this class?
- What is NOT working well for you in this class?
- What more can YOU do to help yourself succeed in this class?
- What more can I do to help you succeed in this class? (Note: “extra credit” is not an option!)
Their answers to #1 or #2 almost always include a tool, service or application. I pay close attention and make adjustments based upon student responses. If a tool is not assisting students, then using that tool is wasted time and money. I need to find what works for them, in addition to what I find valuable.
ASL: You’re a statistician, so you may be a bit biased, but in your opinion, do most teachers utilize student data? Is there a comprehension problem? How do you think we can improve data literacy for the next generation of educators?
BI: In the community college system, nationally, adjunct faculty teach over half of the courses. They do not have the time, the funding, the training, nor the access to student data beyond their course assessments. Both full and part-time faculty teaching online have a bit more student data, depending upon the learning management system they are using. Still, this information is generally limited to online assessments and whether a student clicked on a particular page and possibly for how long that page remained opened.
In my experience, very few faculty, including in the STEM (science, technology, engineering and mathematics) disciplines, utilize student data aside from course assessments. Classroom assessment techniques for f2f classes (see Pat Cross’ work from UC Berkeley) have been studied and promoted for over two decades as very short and easy activities to provide immediate feedback to faculty so that they can address students’ needs in the very next class session. Only a small percentage of faculty regularly use even these techniques.
Since I am a statistician and “data junkie”, I search for the data that will help ME to improve my teaching and support for students’ needs. I use those data to inform the follow-up discussions and revise my own lessons. I do believe that most faculty are not sure what data to utilize or how the data can lead to improvements in student learning. We need to offer short courses for current faculty on data literacy. We need to include data literacy in graduate programs, not just in computer science and statistics programs. The trend toward data literacy and analytics in academia is definitely increasing. We need to ensure that we properly gather and use the data to increase student learning and success.
ASL: What does the future of data-driven teaching look like to you? What changes and innovations would you like to see in the next 1, 5, or 10 years?
BI: Many people in higher education are realizing that high stakes exams are losing their validity as the measure of student knowledge. With the increased use of apps and interactive learning systems, faculty can gather data almost continuously throughout the term, adjusting our teaching to improve student learning. My experience has been that these small assessments with their immediate feedback encourage students to work harder and longer until they succeed. That “harder and longer” is just not in the traditional form that we are used to.
We are witnessing students studying in small chunks of time, fitting their learning in as they wait in line, in-between classes and work, and while their busy lives are continuing on. I expect that the delivery of content will also move into small chunks with more small stakes assessment. It is essential that we, as educators, adapt to the needs of our students.
We also need to find a way to consistently assess prior student learning and veterans’ experiences, so that we can offer modules to give full course credit once the student masters the remaining material. These modules must be individualized, computerized and adapt to various learning styles in order to serve older students’ needs.
Teaching Strategies Q&A: A Stats Professor’s Perspective On Data-Driven Teaching; image attribution flickr user nickwebb; Q&A: How Data Can Make You A Better Teacher